Synopsis:
Motivation: To quantify female reproductive anatomy in MR imaging.
Goal: To develop an AI-based solution to segment the regions of interest (RoIs) for the uterine zone, ovaries, pelvic fluid, and detect benign uterine conditions.
Approach: A deep learning based method is applied on a large representative population of 9334 sagittal T2-weighted female pelvis scans to extract normative menstrual cycle- and aging-curves for various RoIs.
Results: Our proposed normative curves define the standard menstrual cycle and aging trends. RoI segmentation, fibroid, and cyst detection models achieve average foreground dice, specificity and accuracy scores of 83.9%, 95.2% and 94.37%,respectively.
Impact: Proposing a robust, precise AI solution for analyzing female reproductive organs onMR imaging, including uterine zones, ovaries, pelvic fluid, and fibroids/cysts. Using this, we define standard aging and menstrual cycle curves for women.
Introduction:
MR images of the female pelvis provide detailed high resolution images of the reproductive organs which facilitates in-depth analysis of the reproductive system.Nevertheless, ultrasonography stands as the widely accepted imaging modality for assessing female reproductive organs within the medical community and is favored by patients for its ease of access and affordability [1], although some studies demonstrate MRI's superiority in diagnosing certain conditions [1, 2]. Consequently, a significant number of existing AI-driven tools for gynecological analysis have been built for ultrasound modality [3]. Among the limited publications on developingAI-driven tools for female pelvis MRI analysis, most of them focus on malignant conditions or placenta monitoring [4, 5, 6, 7], while a few seek this potential for the general female population [8]. In this work, we proposed a comprehensive AI-driven tool to segment regions of interest (RoIs) for the female reproductive system, namely uterine zones, ovaries, pelvic fluid, and to detect uterine fibroid and cyst conditions(Figure 1). Compared to [8], the proposed solution is more accurate, robust, and efficient by using significantly larger training datasets with more precise ground-truth labels sourced from multiple MRI machines and improving the modeling, e.g., training a unified RoI segmentation network and formulating condition diagnosis as an instance detection task. We have further proposed normative menstrual cycle and aging graphs for a large population of sagittal T2-weighted sequences from a representative sample (N=9334) of adults ranging from age 22 to70 years.
Methods:
Data is acquired from sagittal T2-weighted pelvic sequences obtained as part of a comprehensive preventive whole-body MRI screening protocol. nnU-Net [9] andnn Detection [10] frameworks are used for semantic segmentation and instance detection tasks, respectively. Ground-truth segmentation masks are annotated using ITK-SNAP [11]. One-stage methods take the entire sagittal scan as input, while the two-stage approach restricts the input to a cropped section of the scan. Further details regarding the components of the proposed solution, training sets, and results on validation folds are summarized in Table 1.
Results and Discussions:
In this section, we discuss the elements and results of our proposed solution, considering results on large populations (Figure 2 & 3), hold-out sets (Table 2), andvalidation folds (Table 1).
1) RoI segmentation model segments the uterine zones, ovaries, and pelvic fluid.This enables us to do 2D and 3D measurements over the regions of interest, e.g, measuring the endometrial and junctional zone thickness and quantifying volume of the uterus and ovaries. It further helps with obtaining the input RoIs for the two-stage methods and localizing the conditions. Two settings for training the model are introduced in Table 1. By obtaining the inference results of the model trained with settings 1 (Table 1) over a large population (N=9334),we extracted the normative aging-curves for various RoIs (Figure 2). For the subset of menstrual patients with reported regular menstrual cycles (N=3029),we obtained normative menstrual cycle-curves for ovaries and endometrium(Figure 3). To further enhance the model’s robustness for rare reproductive organ appearances, we queried our radiology report database to extract patients with gynecological conditions. Then, the training dataset was expanded from 188 to 328 samples. These additional samples were manually annotated and consist of cases for which the prior model with settings 1 had subpar performance. The expanded dataset was used to train the RoI segmentation model with settings 2.
2) Uterine cyst model was trained on 227 cases delineated with various types of uterine cysts including Nabothian- and adenomyosis related- cyst in our radiology reports. Compared with the Nabothian cyst model in [8], this model detects cysts in various parts of the uterus which may help with diagnosing more uterine conditions including adenomyosis.
3) Uterine fibroid models are obtained by training nnU-Net [9] and nnDetection [10] on 170 cases reported with uterine fibroid in our report database.
Our nn Detection based fibroid model, as noted in Table 2, significantly improves fibroid classification specificity score (95.2% v.s. 76.19%) compared to the nnU-Net based model, while maintaining a competitive sensitivity. We attribute the performance gain to incorporating instance level information in addition to semantic labels. Both models outperform the solution proposed in[8] as we use a single foreground class with precise ground-truth masks and larger training sample size. In rare cases both models miss degenerated orexophytic fibroids. Also nnDetection predicts the bounding box that fits the fibroid, leading to errors in volume quantification and lower dice scores (Table1). In future, we wish to extend both models to address these issues.
Conclusion:
This study contributes to the advancement of AI-driven tools for MRI analysis in the field of gynecology, enhancing the accuracy and diagnostic capabilities for a broader range of female reproductive conditions. The findings support the potential of MRI as a valuable diagnostic modality in female reproductive health.

Figure 1: Proposed AI-driven method for analyzing female reproductive organs from sagittal T2 pelvis MRI scan.
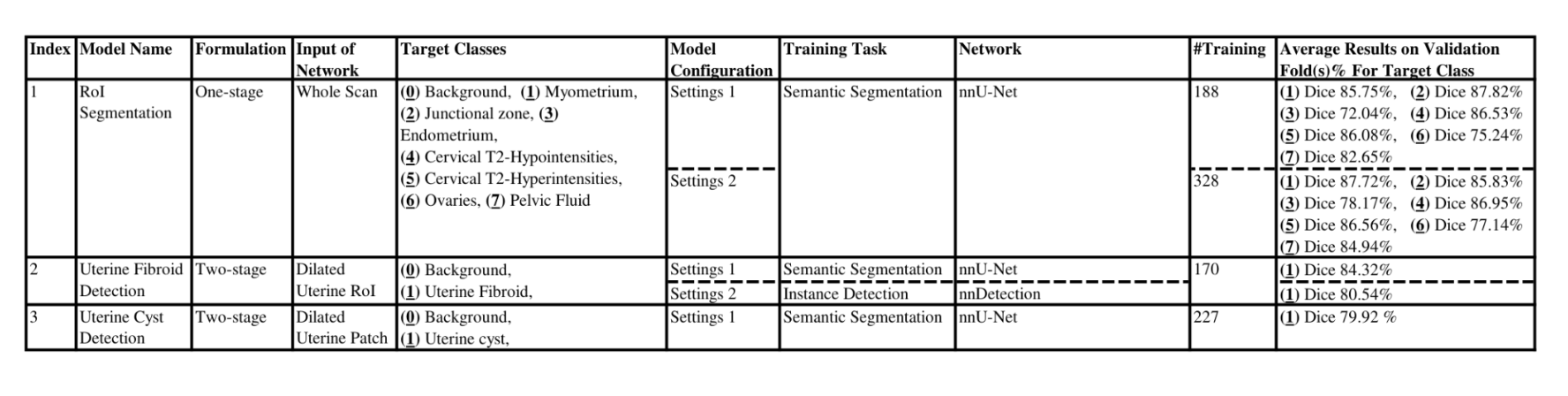
Table 1: Details regarding individual components of the proposed package and training specifics. The term "Dilated Uterine RoI" refers to the RoI achieved by dilating slices of uterine mask for 6 pixels. The "Dilated Uterine patch" is derived by enlarging a 3D-bounding box that fits the organ for 10% in terms of dimension in the sagittal plane. The "#Training" signifies the total number of training and validation samples.Validation is done in a 5-fold cross-validation manner.

Table 2: Evaluation results for the proposed uterine condition diagnosis networks on hold-out test sets. Even though training is formulated as semantic segmentation or instance detection, we report results in terms of classification metrics because the presence of cyst and fibroid conditions is of interest to radiologists .
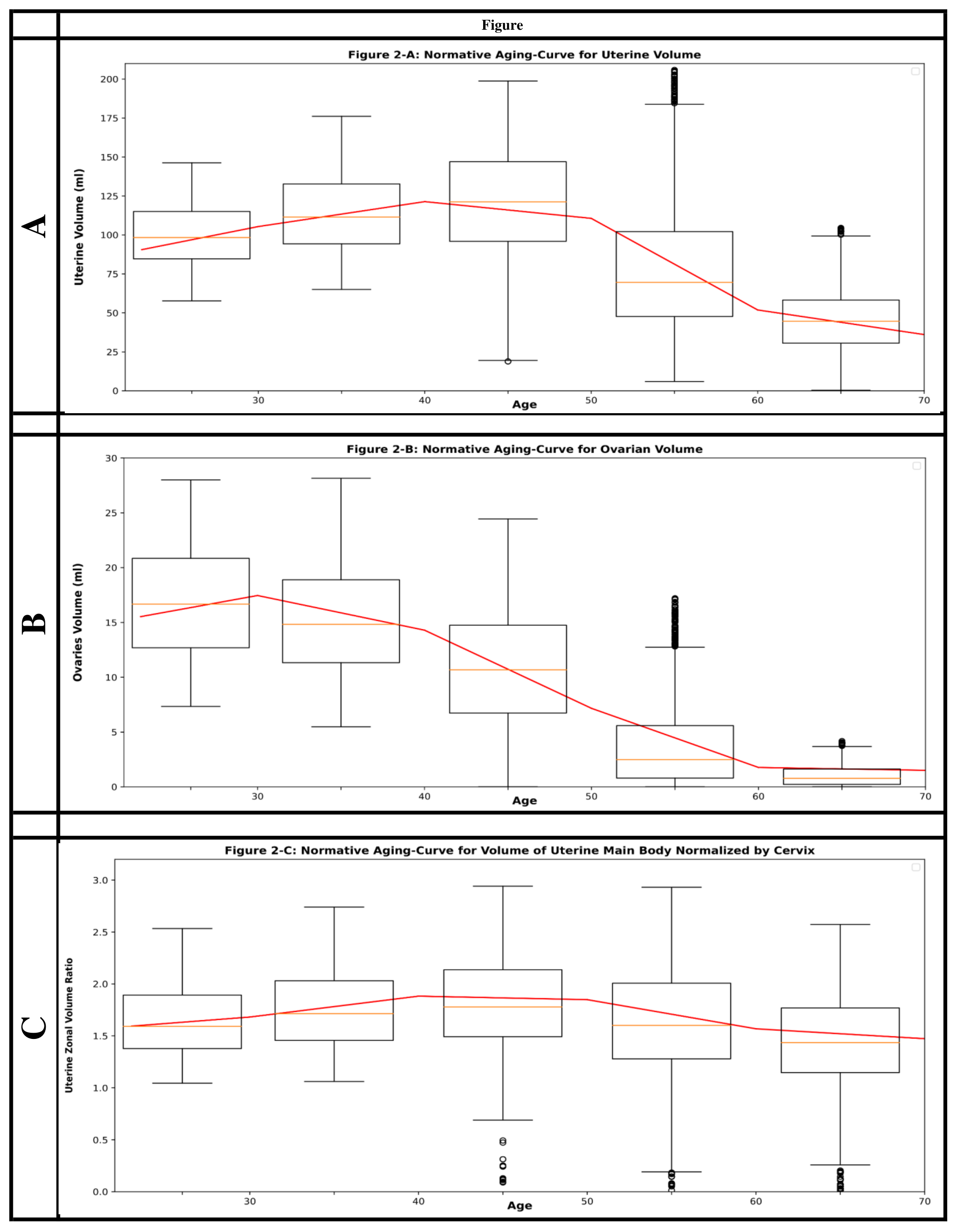
Figure 2: A study on normative aging trends in a large population of N=9334. A)uterine; B) ovarian; and C) uterine zonal volume metrics are shown. Regression analysis is conducted in each decade with distributions visualized using box plots. Our finding for ovaries generally aligns with the prior studies [8, 12], while the ovarian volume increases in the twenties possibly due to the fertility peak. In the future, we plan to study this decade in a larger population.
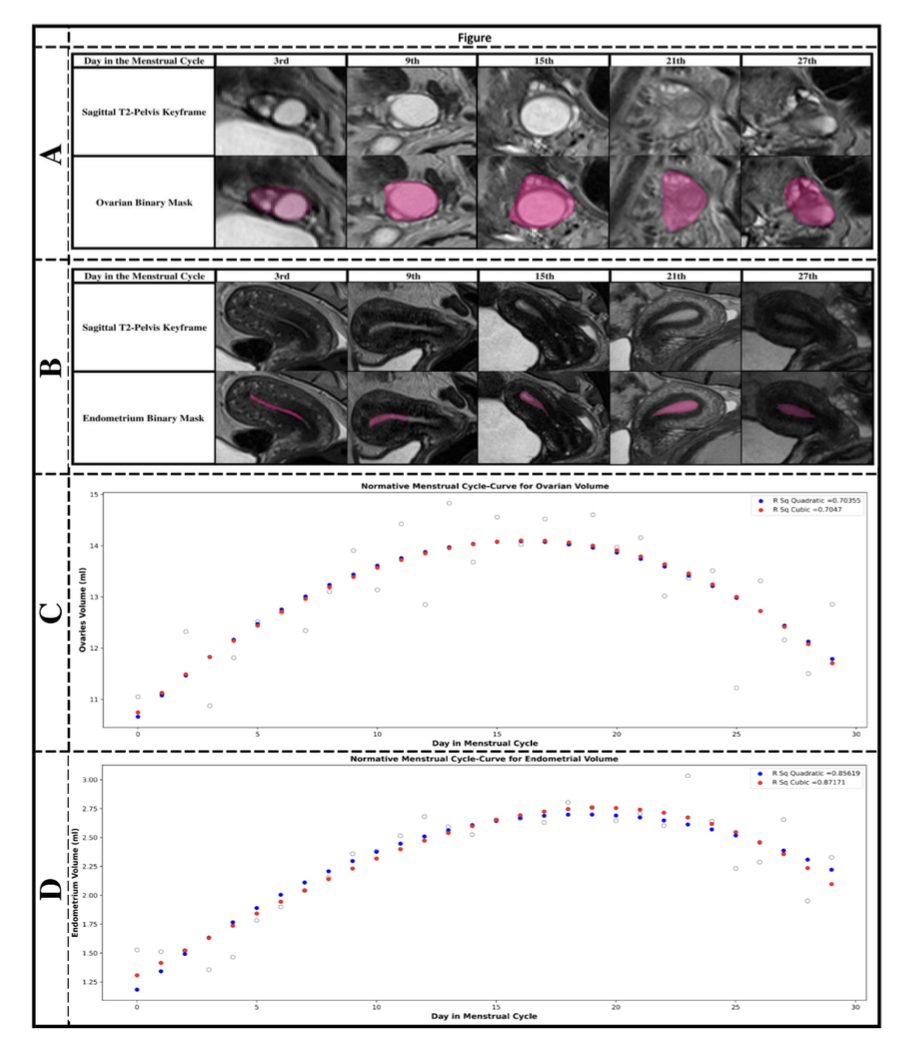
Figure 3: A, B) Examples of ovaries and endometrium for five patients, aged 35, at different menstrual cycle phases. C, D) Normative menstrual cycle-curve for ovariesand endometrium volume. Gray dots show the mean volume for each day while the blue and red points are obtained by fitting quadratic and cubic polynomials to mean values. R-squared values for the fittings are reported. The population is limited to menstrual women reported with regular menstrual cycles , i.e., last menstrual period(LMP) occurring within 28 days of the scan date.
References:
[1]: Hubert, J. and Bergin, D., 2008. Imaging the female pelvis: when should MRI be considered?. Applied radiology, 37(1).
[2] Palmér, M., Åkesson, Å., Marcickiewicz, J., Blank, E., Hogström, L., Torle, M., Mateoiu,C., Dahm-Kähler, P. and Leonhardt, H., 2023. Accuracy of trans vaginal ultrasound versus MRI in the PreOperative Diagnostics of low-grade Endometrial Cancer(PODEC) study: a prospective multi centre study. Clinical radiology, 78(1), pp.70-79.
[3] Chen, Z., Wang, Z., Du, M. and Liu, Z., 2022. Artificial intelligence in the assessment of female reproductive function using ultrasound: A review. Journal of Ultrasound inMedicine, 41(6), pp.1343-1353.
[4]: Shrestha, P., Poudyal, B., Yadollahi, S., Wright, D.E., Gregory, A.V., Warner, J.D.,Korfiatis, P., Green, I.C., Rassier, S.L., Mariani, A. and Kim, B., 2022. A systematic reviewon the use of artificial intelligence in gynecologic imaging–Background, state of the art, and future directions. Gynecologic Oncology.
[5] Urushibara, A., Saida, T., Mori, K., Ishiguro, T., Inoue, K., Masumoto, T., Satoh, T. and Nakajima, T., 2022. The efficacy of deep learning models in the diagnosis of endometrial cancer using MRI: a comparison with radiologists. BMC Medical Imaging,22(1), pp.1-14.
[6] Twickler, D.M., Do, Q.N., Xi, Y., Shahedi, M., Dormer, J., TT, A.D., Lewis, M.A., Spong, C.Y.,Dashe, J.S., Madhuranthakam, A. and Fei, B., 2020. 228: Automated segmentation of the human placenta and uterus with MR imaging using artificial intelligence (AI).American Journal of Obstetrics & Gynecology, 222(1), pp.S158-S159.
[7] Lu, M., Wang, T., Zhu, H. and Li, M., 2023, October. HACL-Net: Hierarchical Attention and Contrastive Learning Network for MRI-Based Placenta Accreta SpectrumDiagnosis. In International Conference on Medical Image Computing andComputer-Assisted Intervention (pp. 304-314). Cham: Springer Nature Switzerland.
[8] Khaghani, J., Basar S., Chodakiewitz Y., London S., Attariwal, R., and Hashemi, S.,2023. A Deep Learning-Based Tool for Analyzing the Female Reproductive System inMR images. International Society for Magnetic Resonance in Medicine (ISMRM), June03-08 2023, Toronto.
[9] Isensee, F., Jaeger, P.F., Kohl, S.A., Petersen, J. and Maier-Hein, K.H., 2021. nnU-Net: a self-configuring method for deep learning-based biomedical image segmentation.Nature methods, 18(2), pp.203-211.
[10] Baumgartner, M., Jäger, P.F., Isensee, F. and Maier-Hein, K.H., 2021. nn Detection: a self-configuring method for medical object detection. In Medical Image Computingand Computer Assisted Intervention–MICCAI 2021: 24th International Conference,Strasbourg, France, September 27–October 1, 2021, Proceedings, Part V 24 (pp.530-539). Springer International Publishing.
[11] Yushkevich, P.A., Piven, J., Hazlett, H.C., Smith, R.G., Ho, S., Gee, J.C. and Gerig, G.,2006. User-guided 3D active contour segmentation of anatomical structures:significantly improved efficiency and reliability. Neuro image, 31(3), pp.1116-1128.
[12] Kelsey, T.W., Dodwell, S.K., Wilkinson, A.G., Greve, T., Andersen, C.Y., Anderson, R.A.and Wallace, W.H.B., 2013. Ovarian volume throughout life: a validated normative model. PloS one, 8(9), p.e71465.